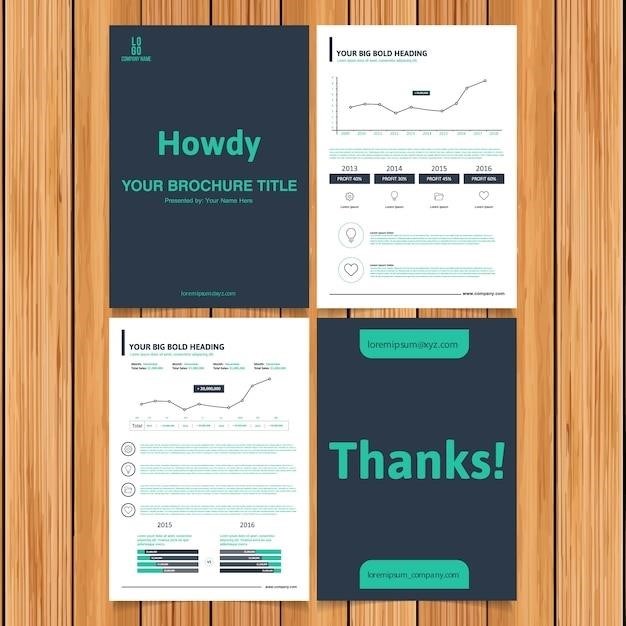
Cauchy Distribution⁚ An Overview
The Cauchy distribution‚ also known as the Lorentzian distribution‚ is a continuous probability distribution. Its probability density function (PDF) is characterized by its heavy tails and lack of defined moments like mean and variance. It finds applications in various fields‚ including physics and statistics.
Defining the Cauchy Probability Density Function (PDF)
The Cauchy probability density function (PDF) describes the probability of a continuous random variable taking on a given value. For a standard Cauchy distribution (location parameter a = 0‚ scale parameter b = 1)‚ the PDF is defined as f(x) = 1/[π(1 + x²)]‚ where x represents the random variable. This function is symmetric around x = 0‚ exhibiting a bell shape but with significantly heavier tails compared to a normal distribution. The heavier tails indicate a higher probability of observing extreme values. The general form‚ incorporating location parameter ‘a’ and scale parameter ‘b’‚ is given by f(x) = [b/π]/[b² + (x ─ a)²]. The scale parameter ‘b’ influences the spread of the distribution‚ while the location parameter ‘a’ shifts the center of the distribution.
The Standard Cauchy Distribution
The standard Cauchy distribution represents a specific case of the Cauchy distribution where both the location parameter (a) and the scale parameter (b) are set to 1 and 0 respectively. This simplification results in a probability density function (PDF) with a particularly straightforward form⁚ f(x) = 1/[π(1 + x²)]. The standard Cauchy distribution is symmetric around its median‚ which is located at x = 0. Unlike many other distributions‚ the standard Cauchy distribution doesn’t possess a defined mean or variance because its tails decay too slowly for these moments to exist. This characteristic makes it a valuable tool for illustrating the limitations of relying solely on mean and variance for describing statistical properties‚ especially when dealing with data containing outliers or extreme values. Its unique properties make it a crucial example in probability theory and statistics.
Parameters⁚ Location and Scale
The general Cauchy distribution is defined by two parameters⁚ the location parameter‚ often denoted as ‘a’‚ and the scale parameter‚ usually represented as ‘b’. The location parameter ‘a’ determines the central tendency of the distribution‚ shifting the entire PDF along the x-axis. It represents the median of the distribution. The scale parameter ‘b’‚ always positive‚ controls the spread or width of the distribution. A larger ‘b’ value leads to a wider‚ flatter distribution‚ while a smaller ‘b’ results in a narrower‚ more peaked distribution. The probability density function (PDF) incorporates these parameters as follows⁚ f(x; a‚ b) = [b / π(b² + (x ─ a)²)]. These parameters provide flexibility in modeling various phenomena where heavy-tailed‚ symmetric distributions are observed.
Properties of the Cauchy Distribution
The Cauchy distribution exhibits distinctive properties‚ including heavy tails‚ a lack of defined moments (mean‚ variance)‚ and a symmetric bell shape. Its unique characteristics set it apart from other probability distributions.
Heavy Tails and Lack of Moments
A defining characteristic of the Cauchy distribution is its exceptionally heavy tails. Unlike the normal distribution where probabilities decay exponentially‚ the Cauchy distribution’s probabilities decrease much more slowly‚ following a polynomial rate. This means that extreme values are far more probable in a Cauchy distribution compared to a normal distribution. Consequently‚ this heavy-tailed behavior leads to a crucial property⁚ the Cauchy distribution lacks defined moments. The mean‚ variance‚ and higher-order moments are undefined because the integrals required to calculate them diverge. This implies that the standard statistical measures used with normal distributions are inapplicable to the Cauchy distribution. The absence of defined moments significantly impacts its behavior and makes it unsuitable for applications where these statistical measures are essential.
Symmetry and Bell Shape
Despite its lack of defined moments‚ the Cauchy distribution exhibits a visually familiar characteristic⁚ a bell shape. Its probability density function (PDF) is symmetric around a central point‚ often referred to as the location parameter. This symmetry is visually apparent when plotting the PDF; it mirrors itself across a vertical line drawn through the central point. However‚ it’s crucial to remember that this visual resemblance to the normal distribution’s bell curve is deceptive. The Cauchy distribution’s heavier tails significantly alter its statistical properties‚ making it distinct from the normal distribution. While both are unimodal (possessing a single peak)‚ the Cauchy’s peak is sharper‚ and its tails extend significantly further‚ impacting the overall probability distribution and the likelihood of observing extreme values.
Relationship to Other Distributions
The Cauchy distribution holds unique connections to other probability distributions. It’s notably linked to the Student’s t-distribution; specifically‚ a Student’s t-distribution with one degree of freedom is identical to the Cauchy distribution. This connection highlights the Cauchy’s role as a limiting case within a family of distributions. Furthermore‚ the ratio of two independent standard normal random variables follows a Cauchy distribution. This relationship provides a way to generate Cauchy-distributed random samples using normally distributed variables. Its connection to the normal distribution‚ despite having drastically different properties‚ is a key aspect of understanding its place within the broader field of probability theory. These relationships facilitate theoretical analyses and offer alternative approaches for modeling and simulation.
Applications of the Cauchy Distribution
The Cauchy distribution finds use in diverse fields. Signal processing‚ particularly in resonance modeling‚ benefits from its unique properties. Statistical modeling employs it for robust estimations‚ while physics and engineering leverage its characteristics in various applications;
Signal Processing and Resonance
In signal processing‚ the Cauchy distribution‚ also known as the Lorentzian distribution‚ plays a significant role in modeling resonance phenomena. Its characteristic bell shape and heavy tails accurately represent the frequency response of resonant systems. The distribution’s parameters‚ particularly the location and scale‚ directly correspond to the resonant frequency and the width of the resonance peak‚ respectively. This makes it an ideal tool for analyzing and characterizing signals exhibiting resonant behavior. Applications include modeling spectral lines in spectroscopy‚ analyzing the response of damped harmonic oscillators‚ and filtering noisy signals that contain resonant components. The heavy tails of the Cauchy distribution are particularly useful in handling outliers and noise that might skew the results of analyses using other distributions. This robustness makes it an attractive option for applications where the signal-to-noise ratio is low or where impulsive noise is present.
Statistical Modeling
While the Cauchy distribution’s lack of defined moments limits its use in some statistical contexts‚ its unique properties make it valuable for specific modeling tasks. Its heavy tails are particularly useful when dealing with datasets containing extreme values or outliers that would disproportionately influence analyses based on distributions with lighter tails‚ such as the normal distribution. For instance‚ in robust regression‚ the Cauchy distribution can provide more reliable estimates of parameters in the presence of outliers‚ as it down-weights their influence compared to the normal distribution. Furthermore‚ its symmetric bell shape and analytic tractability make it suitable for modeling certain types of errors or noise in data where a more robust alternative to the Gaussian model is needed. Its application in modeling financial data‚ where extreme events are common‚ is an active area of research.
Physics and Engineering
In physics and engineering‚ the Cauchy distribution‚ often referred to as the Lorentzian distribution or Breit-Wigner distribution‚ models resonance phenomena. The shape of the distribution accurately reflects the spectral line broadening observed in various physical processes. For example‚ it describes the resonant frequencies of damped oscillators or the energy distribution of particles in certain nuclear reactions. Its heavy tails account for the spread in observed frequencies or energies around the central value. Furthermore‚ the Cauchy distribution arises in the context of random walks and diffusion processes where the step sizes follow a specific power-law distribution. The analytical simplicity of the Cauchy PDF makes it particularly useful in theoretical calculations involving such processes.
Cauchy Distribution vs. Normal Distribution
The Cauchy and normal distributions‚ though both bell-shaped‚ differ significantly. The Cauchy lacks a defined mean and variance‚ unlike the normal distribution‚ and exhibits heavier tails.
Comparison of PDFs
A visual comparison of the probability density functions (PDFs) of the Cauchy and normal distributions reveals striking differences. While both exhibit a bell-shaped curve‚ the Cauchy PDF is more sharply peaked at its center‚ indicating a higher concentration of probability around the median. However‚ the Cauchy distribution’s defining characteristic is its significantly heavier tails. These tails extend much further than those of the normal distribution‚ implying a greater probability of observing extreme values. This contrast directly reflects the fundamental difference in their moments; the normal distribution possesses well-defined mean and variance‚ which are crucial for characterizing its spread and central tendency. In contrast‚ the Cauchy distribution lacks these moments‚ making traditional statistical measures less informative. The heavier tails and lack of defined moments highlight the significant divergence in the behavior of these two distributions‚ even though they share a superficial visual resemblance.
Contrasting Properties
The Cauchy and normal distributions exhibit starkly contrasting properties. The normal distribution is characterized by its well-defined mean and variance‚ allowing for precise quantification of central tendency and dispersion. In contrast‚ the Cauchy distribution lacks both a defined mean and variance; its heavy tails prevent these moments from converging to finite values. This fundamental difference has significant implications for statistical analysis. The normal distribution readily lends itself to the central limit theorem and various statistical tests‚ while the Cauchy distribution’s undefined moments make such applications problematic. Furthermore‚ the Cauchy distribution’s heavier tails imply a greater probability of observing extreme outliers compared to the normal distribution. This makes the Cauchy distribution particularly useful in modeling phenomena prone to extreme events‚ while the normal distribution is better suited for scenarios with relatively less variability around the mean.
Generating Random Samples from the Cauchy Distribution
Generating random samples from the Cauchy distribution can be achieved using the inverse transform sampling method or other advanced sampling techniques.
Inverse Transform Sampling
The inverse transform sampling method offers an elegant approach to generating Cauchy-distributed random numbers. This method leverages the cumulative distribution function (CDF) of the Cauchy distribution. First‚ a random number‚ u‚ is drawn from a uniform distribution between 0 and 1. Then‚ the inverse of the Cauchy CDF is applied to u to obtain a random variate‚ x‚ following the Cauchy distribution. The formula for the inverse CDF is directly derived from the arctangent function inherent in the Cauchy CDF. This technique is computationally efficient and readily implemented in various programming languages. The precision of the generated samples depends on the quality of the underlying uniform random number generator. However‚ the method’s simplicity and directness make it a popular choice for simulating Cauchy random variables.
Other Sampling Methods
Beyond the inverse transform method‚ alternative techniques exist for generating random samples from the Cauchy distribution. Rejection sampling‚ for instance‚ involves generating points from a proposal distribution and accepting them based on a probability determined by the ratio of the Cauchy PDF to the proposal PDF. This method’s efficiency hinges on the choice of the proposal distribution; a well-chosen distribution minimizes wasted samples. Furthermore‚ advanced Markov Chain Monte Carlo (MCMC) methods‚ such as the Metropolis-Hastings algorithm‚ can also be employed. These methods construct a Markov chain whose stationary distribution is the target Cauchy distribution. While MCMC methods can handle more complex scenarios‚ they generally demand higher computational resources compared to the inverse transform method. The selection of the optimal sampling method often depends on the specific application and computational constraints.